Did xAI Mislead About Grok 3’s Benchmarks? OpenAI Disputes Claims
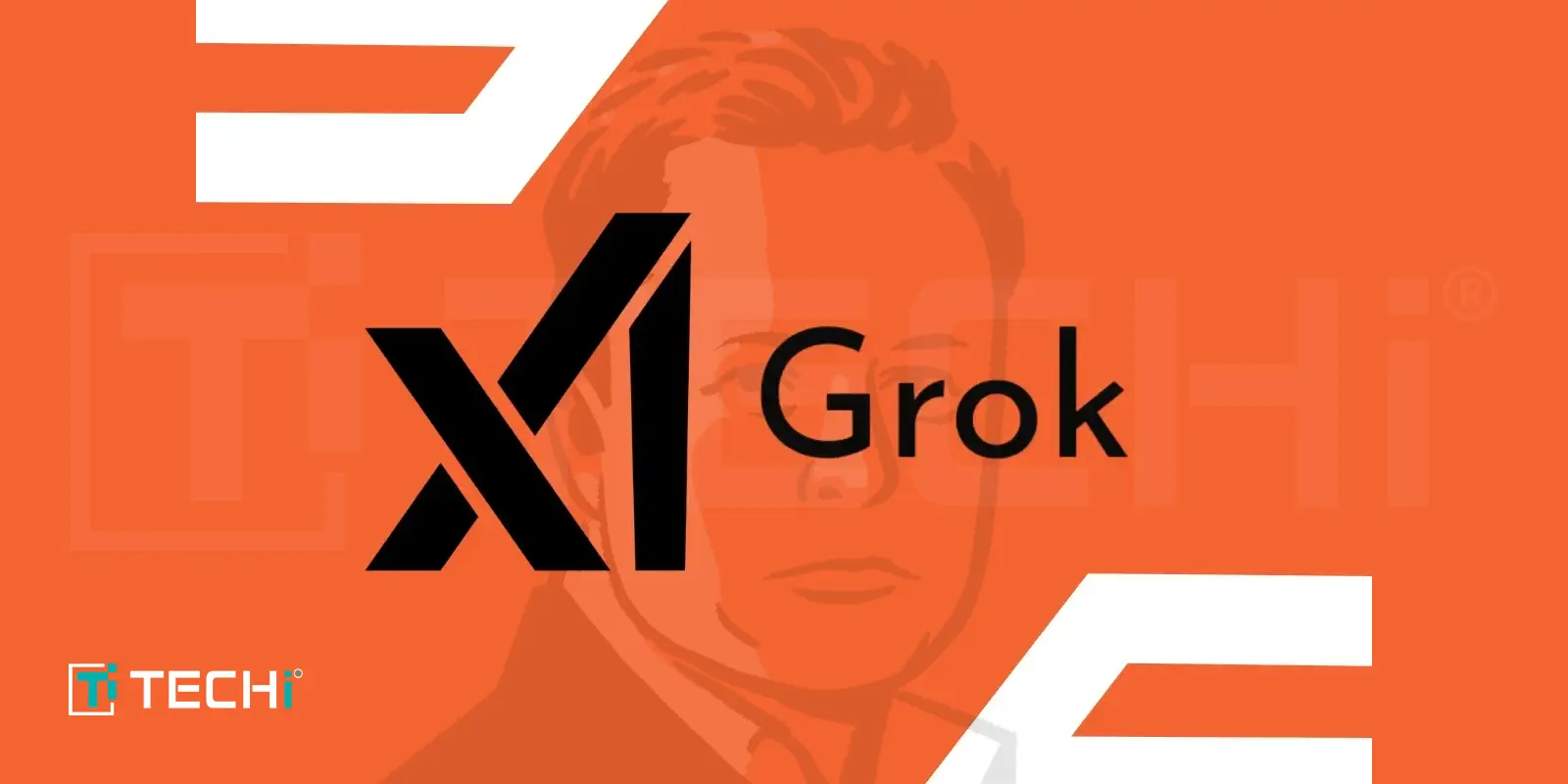
Debates over AI benchmarks have resurfaced following xAI’s recent claims about its latest model, Grok 3. An OpenAI employee publicly accused Elon Musk’s xAI of presenting misleading benchmark results, while xAI co-founder Igor Babushkin defended the company’s methodology. The controversy stems from a graph published by xAI showing Grok3 performance on AIME 2025, a benchmark based on complex mathematical problems. While some AI researchers question AIME’s validity as an AI benchmark, it remains a commonly used test for assessing AI models’ math capabilities.
The Missing Benchmark Data
In xAI’s chart, Grok3 Reasoning Beta and Grok3 mini Reasoning were shown to outperform OpenAI’s o3-mini-high model on AIME 2025. However, OpenAI employees quickly pointed out that xAI did not include o3-mini-high’s score at “cons@64.” The “cons@64” (consensus@64) metric allows a model to attempt each problem 64 times, selecting the most frequent response as the final answer. Since this significantly improves a model’s benchmark scores, omitting it from xAI’s comparison may have made Grok 3 appear more advanced than it actually is.
When comparing @1 scores (which measure a model’s first attempt accuracy), Grok 3 Reasoning Beta and Grok 3 mini Reasoning scored below OpenAI’s o3-mini-high. Additionally, Grok 3 Reasoning Beta trailed behind OpenAI’s o1 model set to “medium” computing, raising further questions about xAI’s claim that Grok 3 is the “world’s smartest AI.”
xAI Defends Its Approach, OpenAI Calls for Transparency
Igor Babushkin, co-founder of xAI, responded on X, arguing that OpenAI has also presented selective benchmarks, though mainly when comparing its models. A third-party AI researcher attempted to provide a more balanced view by compiling a graph displaying various models’ performance at cons@64, aiming to offer a more transparent comparison. However, AI researcher Nathan Lambert pointed out a key missing element in the debate: computational cost. Without knowing how much computational power (and cost) was required for each model to achieve its best scores, benchmarking alone does not fully convey an AI model’s efficiency or real-world capabilities.
What’s Next for AI Benchmarks?
The dispute between xAI and OpenAI highlights ongoing challenges in AI benchmarking. As AI labs race to demonstrate superiority, the lack of standardized, transparent, and cost-aware metrics continues to fuel debates over how AI models should be evaluated. While xAI stands by its claims, OpenAI’s criticism raises questions about how AI companies should present performance results to avoid misleading comparisons. The broader AI community may need to push for more standardized evaluation methods to ensure fairness and accuracy in future AI model comparisons.
Read More: Nvidia CEO Jensen Huang says market got it wrong about DeepSeek’s impact